Market Situation
Predictive analytics and maintenance are becoming increasingly important in the wind renewable industry, as operators seek to optimize performance, reduce costs, and increase efficiency. The market for these solutions is growing, driven by the recognition of their value in improving turbine maintenance and reducing downtime. Furthermore, the integration with other emerging technologies, such as IoT and cloud computing, is creating new opportunities for centralized management and decision-making, and enhancing operational efficiency across multiple turbines and wind farms. As the wind renewable industry continues to develop and mature, predictive analytics and maintenance will undoubtedly play a critical role in shaping its future.
Key Benefit
The key benefit of a predictive analytics solution for wind asset owners is the ability to optimize asset performance, reduce costs, and improve operational efficiency, by leveraging data-driven insights and proactive maintenance strategies. Asset owners can achieve higher energy production, extend asset lifespan, ensure safety and reliability, and make informed decisions to maximize the return on their wind energy investments.
The Solution
GPM Horizon’s Predictive Feature
GPM Horizon is designed to help the wind energy operators, maintenance personnel, asset managers and other users to quickly identify issues with their wind assets and take appropriate action, thus reducing downtime and improving overall performance, which increases efficiency, productivity, and profitability.
In the GPM Horizon Predictive module, we have created artificial intelligence algorithms designed to forecast failures and outages by analyzing abnormalities in vibration, spectra, and SCADA data. The solution has already served to identify possible damages early, leading to significant money savings for our clients.
Key Differentiator of GPM Horizon’s Predictive module
The predictive module provides in-depth analytics, presents the results in an understandable manner, and simultaneously learns from user inputs.
Asset failure suggestions:
The solution suggests assets that are likely to fail, saving time by focusing analysis on those assets that would cause potential issues, rather than focusing on every asset.
Specific suggestions for potential failures:
The solution identifies the potential failures that need to be addressed and offers explicit recommendations on what to inspect for potential repairs or replacements.
Improvement of failure asset and specificity:
Based on user feedback, the algorithm strengthens the specificity of the proposed failures and increases the accuracy of asset failure identification in the future.
Key Benefits of the GPM Horizon Predictive Module
The predictive module of GPM Horizon was designed by asset managers, for asset managers, and offers numerous benefits:
- Enhanced asset performance: The solution can identify patterns, abnormalities, and probable failures by analyzing historical and current data. This allows clients to proactively address issues, make informed decisions, and take preventive measures to maximize energy production and the overall performance of individual assets and the entire portfolio.
- Improved maintenance efficiency: Users have the option to switch from conventional, time-based maintenance methods to condition-based or preventive maintenance techniques. The solution reduces wasteful maintenance tasks and maximizes resource allocation, by detecting maintenance needs. This results in more effective maintenance, less downtime, and reduced costs.
- Cost reduction: The solution saves costs and significantly boosts the ROI by identifying unplanned downtime and significant potential equipment failures.
- Extended asset lifespan: The solution increases the lifespan of wind assets by enabling early diagnosis of possible problems and proactive maintenance. This results in a reduced need for early asset replacement and capital expenditure.
- Operational effectiveness: Predictive analytics’ insights enable users to make data-driven choices for operational optimization. Users can take corrective action to increase energy output, lower losses, and enhance overall operational efficiency by detecting performance inefficiencies, underperforming assets, or suboptimal operational settings.
- Increased dependability and safety: The solution also aids in identifying and addressing potential safety hazards in wind assets. The system notifies users of unusual circumstances that could jeopardize safety, by spotting anomalies in data connected to SCADA, vibration, or spectrum.
- Reporting: Through logical dashboards and reports, users can access the most recent data on asset performance, health, and maintenance requirements. This facilitates prompt decision-making, effective stakeholder communication, and efficient administration of wind assets.
How does the GPM Horizon Predictive module work?
The Predictive module works in the following steps:
Step 1: Identifying Anomalies
The neural network (AI) is “trained” with the data that was received and “learns” what a normal operation modus is. Anomalies can then be detected against this “normal” situation using the developed algorithms if a defined threshold is exceeded.
Step 2: Transition from Anomaly to Failure Mode
Anomalies are fed into a FMEA Matrix (failure mode and effects analysis) developed by combining the know-how of data scientists, wind turbine technology specialists and wind farm operations experts.
-The FMEA Matrix is an automatized decision tree that provides the most probable failure modes for each anomaly or anomaly groups.
-Failure modes represent the machinery breakdown or operational constraint which might derive from the detected anomalies.
Step 3: AI results are enriched with expert knowledge
Wind turbine operation experts discuss the findings and recommendations of the AI algorithms and evaluate them based on their know-how and experience. These findings are filtered according to the experts’ evaluation on plausibility, severity, and urgency. This process minimizes false positives and enriches the algorithms findings and recommendations with experts’ know-how.
Step 4: Feedback Loops
Early failure identification is made possible by AI algorithms when combined with technical know-how and operational experience. This helps prevent costly machinery breakdowns and the ensuing production losses.
User Experience with the GPM Horizon Predictive Module
A dashboard presents users with all detected anomalies, classified by severity, including:
- Red: Alert (immediate action required).
- Orange: Notification (check during next maintenance).
- Yellow: Watchlist (no action required at the moment).You can filter by severity, anomaly type (SCADA, spectra, vibration), sensor, status, and user action.
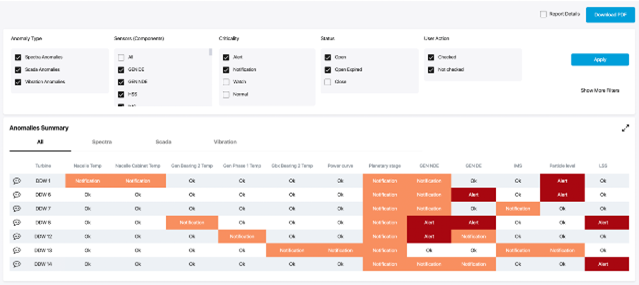
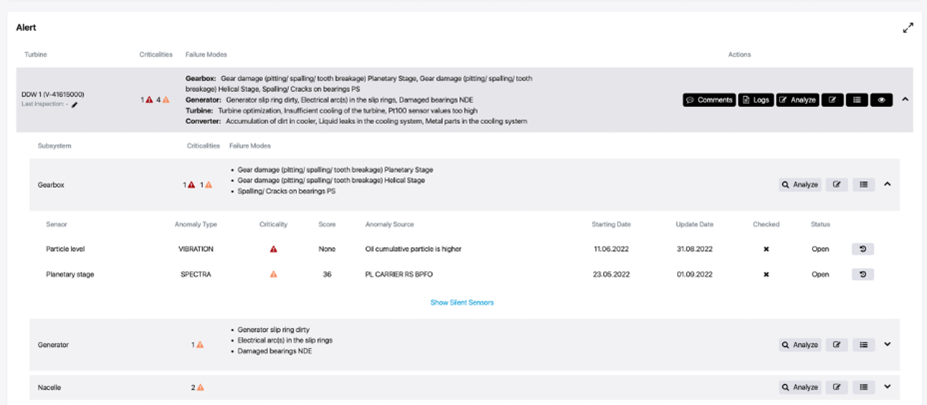
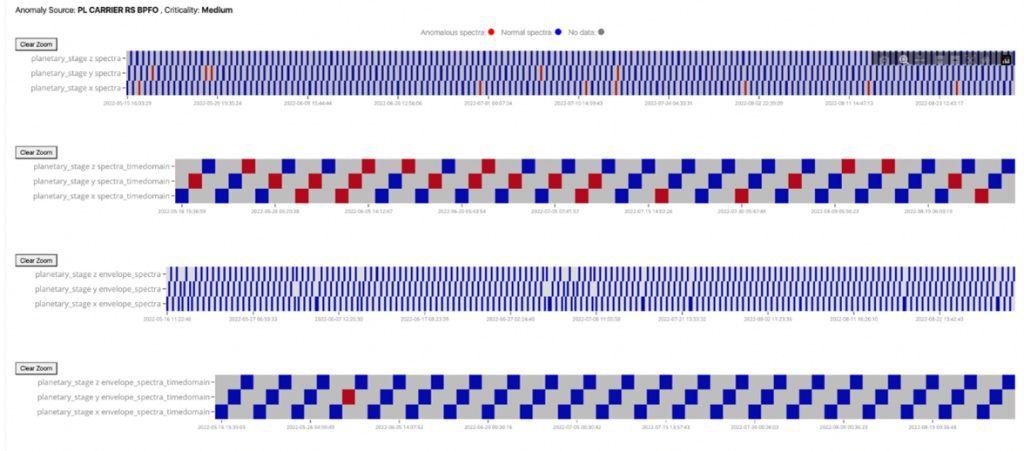
zooming in and out on the time series.
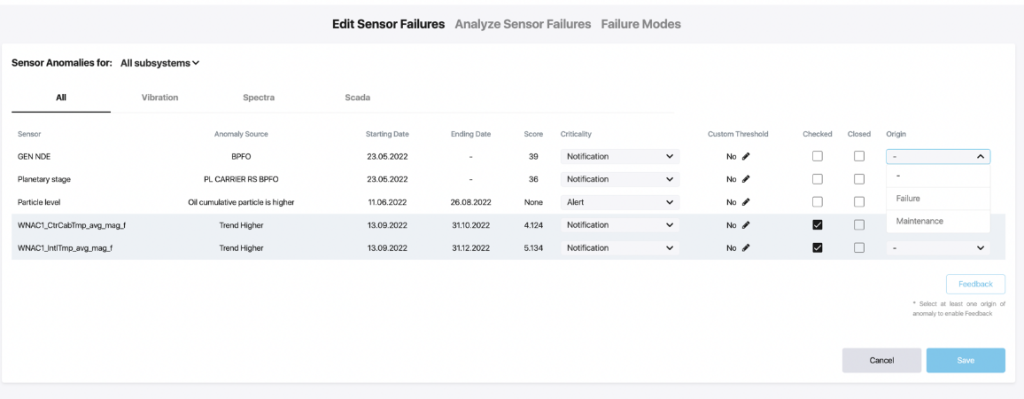
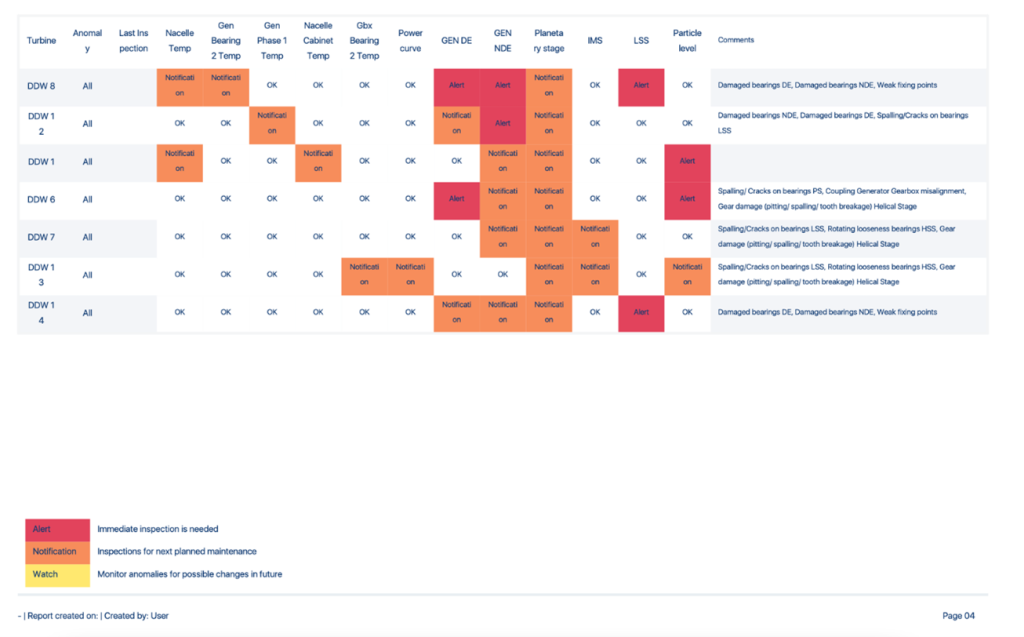
In conclusion, the use of predictive analytics in wind asset management offers both challenges and opportunities. While it holds the potential to improve operational efficiency, reduce downtime, and optimize maintenance strategies, several challenges need to be addressed for its successful implementation.
Data quality and availability pose significant challenges, including inconsistencies, missing values, and limitations in data collection. Establishing robust data collection processes, data cleansing Techniques and reliable communication protocols are essential to overcome these challenges
The volume and velocity of data generated by wind assets require efficient processing and analysis. Leveraging scalable cloud infrastructure, optimized algorithms, and data compression techniques can help handle the high data volume and velocity. Algorithm selection and calibration can be complex due to the influence of asset types, environmental conditions, and operational contexts. Careful evaluation, comparison, and fine-tuning of algorithms are necessary to ensure they capture relevant patterns and anomalies.
Model training and validation require sufficient and diverse training data. Accurate data collection, labeling, and validation processes are crucial to develop reliable predictive models that can handle a wide range of operating conditions.
Interpretability of predictive analytics models is essential for gaining trust from operators and stakeholders. Techniques such as feature importance analysis and model methods can enhance the interpretability of the results. GPM’s experts are aware of these challenges and work to address them every day. With each update, we move towards solving them completely.
Despite these challenges, there are significant opportunities in using cloud-based predictive analytics solution for centralized management of wind assets. It enables proactive maintenance by predicting and addressing potential failures before they occur. It also optimizes maintenance schedules, reducing downtime and increasing operational efficiency. Predictive analytics can improve resource allocation, minimize costs, and maximize the lifespan of wind assets.
Moreover, predictive analytics provides valuable insights into asset performance, allowing for continuous improvement in design and operation. It supports data-driven decision-making, enabling operators to make informed choices based on accurate predictions and real-time monitoring.
GPM experts are committed to addressing these challenges by leveraging the opportunities in the application of predictive analytics in wind asset management. We believe this can revolutionize the industry, leading to increased reliability, reduced costs, and enhanced sustainability in wind energy generation.