Disclaimer: the details and outcomes described in this article are based on a presentation prepared by GPM&S in collaboration with Arowya (BKW Energie AG) for Wind Europe 2024 event.
As we witness a shift towards sustainability in the energy sector, renewable energy assets are becoming increasingly vital in the global energy landscape. However, this transition presents unique challenges, especially in asset management, performance monitoring, and maintenance.
At GPM, we’re at the forefront of this change, leveraging artificial intelligence (AI) to transform renewable energy asset management. Through our collaboration with Arowya (BKW Energie AG), we’re pioneering the integration of advanced Predictive Maintenance Analytics (PMA) into energy asset management practices, merging AI precision with human insights.
At the heart of our PMA system lies a synergistic fusion of AI technologies and human expertise. This approach allows us to proactively identify potential issues and optimize maintenance processes for renewable energy assets, thereby increasing their lifespan and reducing operational expenses.
A comprehensive, four-part approach to predictive maintenance
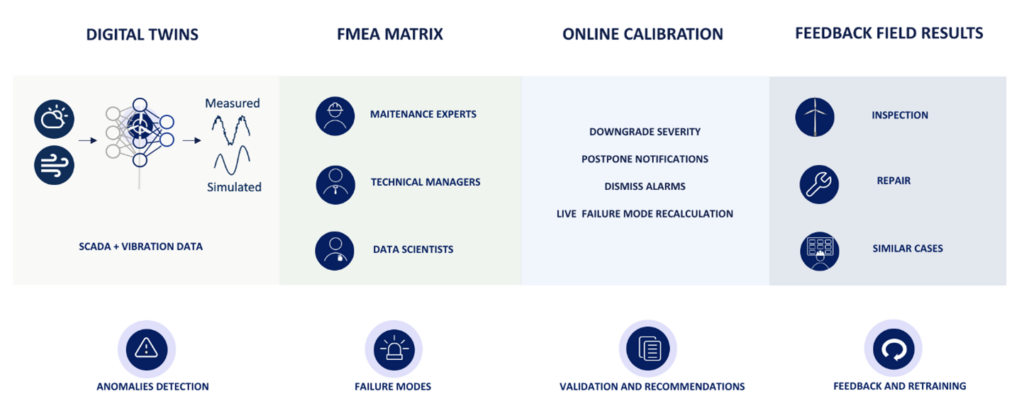
Our innovative PMA system is built on four foundational components that create a robust, closed-loop process for predictive maintenance:
- Machine Learning models: the first pillar of our PMA system are sophisticated Machine Learning (ML) models, particularly deep learning-based digital twins. These models provide a consistent approach to anomaly detection and severity assessment, enabling a comprehensive analysis of asset performance in real-time.
- Digital Twins are virtual replicas of physical assets, developed to mirror the real-time status, working condition, and behavior of wind turbines. By simulating the expected operational output based on historical and real-time data from both Supervisory Control and Data Acquisition (SCADA) and Condition Monitoring Systems (CMS), these models can detect deviations indicative of potential issues, long before they might cause considerable damage or downtime.
- Data Integration and analysis of vast datasets from various sources, including the SCADA and the CMS, allow our ML models to learn from a broad spectrum of operational scenarios. This comprehensive data input ensures a nuanced understanding of normal versus anomalous operational patterns, enhancing the predictive accuracy of our models.
- Failure Mode and Effect Analysis (FMEA) matrix: The second pillar emphasizes the integration of human expertise through the development of a Failure Mode and Effect Analysis (FMEA) matrix. This matrix, a collaborative creation of wind turbine maintenance experts, technical managers, and data scientists, bridges the gap between AI predictions and practical, actionable insights.
- Collaborative Development: the FMEA matrix is not a static tool but a living document that evolves through continuous collaboration between field experts and data scientists. This ensures that the matrix remains relevant and accurate, reflecting the latest in technological advancements and maintenance practices.
- Probable Failure Modes Prediction: beyond mere anomaly detection, the FMEA matrix, supplemented by an additional AI model, aids in pinpointing the most probable failure modes. This predictive capability allows maintenance teams to prioritize their efforts, focusing on the most critical issues identified by a blend of AI insights and expert analysis.
- Online calibration: The third pillar, online calibration, is pivotal in maintaining the relevancy and accuracy of the PMA system. This component facilitates a dynamic interaction between the AI models and human operators, allowing for real-time adjustments based on expert feedback and validation.
- Interactive Feedback Mechanism: the system’s design incorporates mechanisms for experts to validate, dismiss, or downgrade detected anomalies. This feedback directly influences the AI models, enabling them to adjust predictions and recommendations based on human expertise.
- Threshold Adjustments and Validation: the calibration process also includes adjusting anomaly detection thresholds and validating the most probable failure modes. This ensures that the system remains sensitive to the peculiarities of asset behavior, avoiding false alarms while promptly identifying genuine concerns.
- Feedback loop and model retraining: The final pillar completes the predictive maintenance cycle, integrating feedback from onsite inspections and maintenance reports back into the ML models. This feedback loop is essential for the iterative improvement of the system’s predictive capabilities.
- Retraining with Real-World Data: post-maintenance feedback is utilized to retrain the ML models, incorporating new data and insights gathered from the field. This process ensures that the models remain up to date with the latest operational realities, enhancing their predictive accuracy over time.
- Anonymized Case Study Database: the accumulation of maintenance reports and feedback forms a valuable database of anonymized case studies. This repository not only aids in refining the AI models, but also serves as a resource for identifying patterns and trends across different assets and conditions.
Demonstrating value: real-world applications
In our Wind Europe 2024 presentation, we share a real-life scenario where our anomaly detection system detected an unusual rise in generator stator and bearing temperatures. This early detection triggered a series of analyses and actions, showcasing the effectiveness of the PMA system.
Utilizing the anomaly detection, the system’s FMEA matrix indicated a probable issue with the cooling system’s fan, informed by sophisticated analysis of historical data, machine learning insights, and human expertise. The specific identification of a cooling system issue demonstrates the system’s precision in diagnosing potential failures, allowing for targeted inspections and maintenance actions.
A physical inspection validated our diagnosis, leading to the replacement of the faulty generator fan. This swift action averted further complications, underscoring the operational advantages of the PMA system and its ability to preemptively address issues, resulting in significant cost savings. Upon resolving the anomaly, the maintenance team’s findings were integrated back into the system, refining predictive models for increased accuracy in the future. This feedback loop not only enhances predictive capabilities but also enriches the anonymized case study database, facilitating pattern recognition and enhancing diagnostic accuracy.
Looking forward: expanding the Horizon
We aim to elevate human expertise within our PMA system while automating routine tasks. By integrating with CMMS for automatic feedback and expanding our case study database, we aim to minimize manual intervention and adopt a more prescriptive maintenance model. This evolution not only optimizes maintenance schedules and minimizes operational disruptions but also redefines risk management and could inspire the development of tailored insurance products for the renewable energy sector.
Our flagship product, GPM Horizon, already features a Predictive module with comprehensive capabilities. And the exciting news is that our list of predictive features continues to grow quarter by quarter.
Our journey with the Predictive Maintenance Analytics system demonstrates the transformative impact of fusing AI with human expertise in the renewable energy sector. As we refine our approach and embrace technological advancements, we not only enhance the performance and reliability of renewable energy assets but also pave the way for a more sustainable and efficient future in energy management.